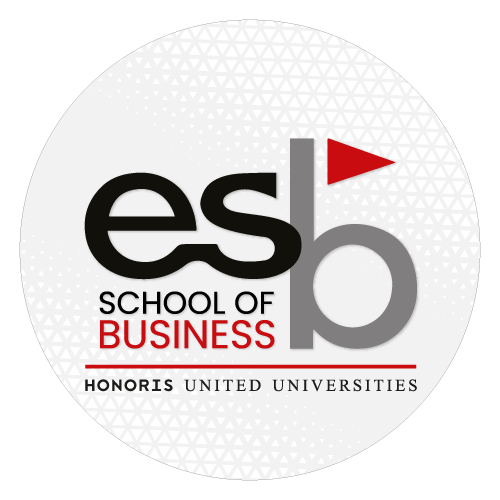
Campus ESB Lot N° 46, Z.I Chotrana II, 2088, Ariana, Tunisia
Email : contact.esb@esprit.tn Phone : +(216) 70 168 700

The first day of the workshop (plenary sessions) will be a hybrid meeting at ESPRIT (Bloc L auditorium) and online for remote participants and attendees.
The second day of the workshop (NVIDIA DLI Instructor-led with certification) will be conducted in a physical face-to-face format at ESPRIT (Bloc A auditorium). Webex link : ieeemeetings.webex.com
Free registration: To attend, please register by completing this form. Please note that limited seats are available, Make sure to register at the earliest.
In contrast to discriminative AI techniques that usually aim to classify data or to distinguish things, Generative AI models are mostly based on unsupervised algorithms capable of learning the underlying representation associated with input data and using it to generate brand-new, synthetic (yet realistic!) content that preserves a similarity to the original data.
While the term “Generative AI” has often been associated with deep fakes, it has proven itself as a potential driver for creativity, innovation, and digital business transformation. The MIT Technology Review described Generative AI models as one of the most promising advances in the world of AI in the past decade. Gartner has also listed Generative AI among the Top 12 technology trends to watch out in 2022 and beyond.
Generative AI approaches present enormous opportunities to drive innovation across many fields and industries: Healthcare, art, media & entertainment, marketing, manufacturing, automotive, robotics, pharmaceutical, security, aerospace, journalism, defense, and software development to name a few.
As Generative AI continues to stimulate our creativity and to positively affect our lives, it raises serious ethical and legal challenges regarding its potential misuse to commit misleading and deceptive conduct or fraud.
The aim of this workshop is to provide a forum for academic researchers, R&D professionals, PhD candidates, leading data science experts, entrepreneurs, and interested parties to share recent research developments and latest solutions pertaining to Generative AI models and applications, their use cases, the underlying challenges and future research directions in this emerging area. The workshop will be carried out through presentations, open discussions, exchange of ideas among researchers and practitioners and through practical hands-on training sessions.
The main desired outcomes of this workshop consist of:
(1) contributing towards capacity building in the field of Generative AI.
(2) raising awareness about the potential role Generative AI can play in business innovation, digital transformation, and value creation.
(3) engaging the audience to reflect upon the ethical, legal, and societal issue underlying the usage Generative AI, and
(4) seeding collaborations and promoting the sharing of ideas, and perspectives in this area.
The event is structured around four main themes:
The workshop will be organized in two days:
Day 2
Duration: 8 hours
Location: ESPRIT School of Engineering, Bloc E.
Instructor: Nehla DABBEBI
Certificate: Upon successful completion of the assessment, participants will receive a Deep Learning Institute (DLI) certificate to recognize their subject matter competency and support professional career growth.
Learning objectives:
By participating in this workshop, you’ll:
Prerequisites:
Suggested materials to satisfy prerequisites: Getting Started with Deep Learning, Intro to Machine Learning
Technologies: NVIDIA RAPIDS™, XGBoost, TensorFlow, Keras, pandas, autoencoders, GANs
Hardware Requirements: Desktop or laptop computer capable of running the latest version of Chrome or Firefox. Each participant will be provided with dedicated access to a fully configured, GPU-accelerated server in the cloud.
Language: French / English
Workshop datasheet: https://nvdam.widen.net/s/n9jm9fjwfx/dli—anomaly-detection-datasheet-update–1811800-r3-1
ABSTRACT:
Generative artificial intelligence (G-AI) – widely known as the creative side of AI- is a disruptive technology that is playing today a central role in content creation. G-AI involves the detection of the underlying pattern associated with existing data (text, images, audios files or videos) to generate new, yet realistic artifacts that reflect the key features of the original training data.
In this talk, I will provide an overview of the present state of the key use and mis-use cases of Generative AI (G-AI) with the aim to stimulate innovation, entrepreneurship, and future research around this promising AI field, while drawing attention to the potential malicious uses and abuses of G-AI by fraudulent people and cybercriminals.
The presentation will not provide an exhaustive coverage of all use-cases, but will try to expose key applications across a variety of domains: Data augmentation, Arts, Media & Entertainment, Marketing, Manufacturing & new product design, Healthcare, Drug discovery, Pharmaceutical, Metaverse, Software development, Image processing, Security, Banking and investment,
I will also try to shed light on the challenges and limitations of current G-AI approaches, while trying to sketch a “fuzzy” view for the road ahead for G-AI. More…
ABSTRACT:
In this talk, I will present an overview of the state of the art in Generative AI (G-AI) models and approaches.
After providing a brief historical perspective on the emergence of the G-AI field, I will address four key G-AI models, namely Generative Adversarial Network (GAN), Transformer-based models, Autoregressive Convolutional Neural Networks (AR-CNNs), and Variational Autoencoders (VAEs). For each G-AI model, I will highlight its general approach, distinctive features, limitations and key application areas. More…
ABSTRACT:
The ability to generate candidate molecules with certain chemical properties is key in novel material discovery. The generation capability has been improved using larger training data, sophisticated generative models, and sampling techniques. However, the multi-level evaluation of generative models for material discovery and the characterization of the generated candidate molecules is not extensively studied in the state of the art. Such evaluations help to improve understanding of the generative process, differentiate across models, and facilitate interaction between machine learning researchers and materials scientists. In this talk, we present our toolkit for Multi-level Performance Evaluation of Generative mOdels (MPEGO) for material discovery applications. MPEGO aims to hierarchically characterize and quantify the capability of generative models across the chemical and biological properties of molecules. MPEGO is model agnostic, and it can be integrated with any generative models for material discovery and beyond. More…
ABSTRACT:
Deep generative models, such as Variational Autoencoders (VAEs) and Generative Adversarial Networks (GANs), have been employed widely in computational creativity research. However, such models discourage out-of-distribution generation to avoid spurious sample generation, thereby limiting their creativity. Thus, incorporating research on human creativity into generative deep learning techniques presents an opportunity to make their outputs more compelling and human-like. As we see the emergence of generative models directed toward creativity research, a need for machine learning-based surrogate metrics to characterize creative output from these models is imperative. In this talk, we will discuss a method based on subset scanning to identify and characterize creative processes by detecting a subset of anomalous node activations in the hidden layers of the generative models. More…
ABSTRACT:
Adversarial attacks can disrupt any AI/ML based system functionalities; while handling such attacks are challenging, but also provide significant research opportunities. This talk will cover emerging adversarial machine learning (AML) attacks on systems and the state-of-the-art defense techniques. First, I will discuss how and where adversarial attacks can happen in an AI/ML model and framework. I will then present classification of adversarial attacks and their severity and applicability in real-world problems and what steps can be taken to mitigate their effects. The role of GAN in in adversarial attacks and as a defense strategy. I will discuss a dual-filtering strategy could mitigate adaptive or advanced adversarial manipulations for wide-range of ML attacks with higher accuracy. The developed dual-filter software can be used as a wrapper to any existing ML-based decision support system to prevent a wide variety of adversarial evasion attacks. The DF framework utilizes two set of filters based on positive (input filters) and negative (output filters) verification strategies that can communicate with each other for higher robustness. More…
ABSTRACT:
Artificial Intelligence (AI) has become a reality for almost all types of businesses and societies. However, trust in AI is something that is challenging its full adoption. That is particularly because the design, development, deployment, and use of AI, if not done according to clear principles and sound practices, may lead to risks to individuals, groups, and even society. Generative AI is an area where trustworthiness may be even more challenging.
From the business standpoint, to mitigate the risks that Generative AI products and services could infringe upon fundamental rights of the individuals and groups subjected to them, organizations would be better positioned if they have a good understanding of the legal and ethical considerations related to the adoption of those products and services. The intent of this contribution is to discuss the legal and ethical perspectives related to the adoption of Generative AI. More…
ABSTRACT:
GT4SD is a platform developed by IBM Research to accelerate hypothesis generation in the scientific discovery process. It provides a library for making state-of-the-art generative AI models easier to use. Users can perform inference to generate new hypotheses and retrain the models with custom data sets to tune results to specific domains. It serves a broad range of applications from materials science to drug discovery and natural language and is compatible with most popular deep learning frameworks: Pytorch, Pytorch lightning, HuggingFace Transformers, GuacaMol, TorchDrug and Moses, among others.
For AI/ML practitioners developing new generative models, GT4SD’s common framework makes models easily accessible to a broader community, who can deploy with just a few lines of code. And for scientists and students interested in using generative models in their scientific research, GT4SD offers a centralized environment to access and try out a number of different models— all of which are pretrained— as well as simplification of model use through consistent commands for inferencing or retraining with default parameter setting.
For materials discovery and drug discovery scientists in particular, GT4SD provides models that can generate new molecule designs based on properties such as target proteins, target omics profiles, scaffolds distances, binding energies and more. These models can be retrained with users’ own data on molecule structures and properties to build domain-specific intelligence. The AI-generated molecule designs can accelerate expert knowledge by replacing manual processes and reducing human bias in the discovery process. More…
Powered by Same Team