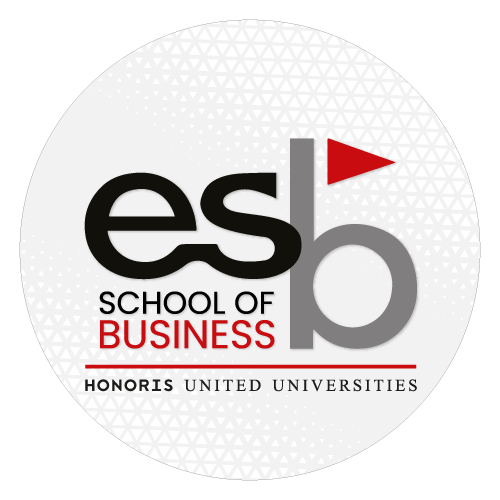
Campus ESB Lot N° 46, Z.I Chotrana II, 2088, Ariana, Tunisia
Email : contact.esb@esprit.tn Tél : +(216) 70 168 700

We are delighted to announce the 2nd Edition of the Workshop on Emerging ICT Trends & Applications (WEITA 2025), dedicated to the theme:
This highly anticipated event is proudly organized by ESPRIT—encompassing the School of Business, the School of Engineering, and ESPRIM—in collaboration with the IEEE Tunisia , EMSI Morocco and IEEE Student Branch.
📅 Dates Wednesday and Thursday, February 12–13, 2025.
Artificial Intelligence (AI) systems have become an integral part of modern society, with their deployment spanning numerous critical applications such as healthcare, finance, energy, transportation, cybersecurity, justice systems, and manufacturing.
Many of these AI-driven systems are designed to assist in or directly make decisions that can have far-reaching implications. Moreover, the reliance on specialized hardware accelerators for AI workloads introduces the risk of hardware faults, which can pose significant challenges, particularly in safety-critical applications where accuracy and reliability cannot be compromised.
These challenges underscore the urgent need for AI models that are not only accurate but also explainable and interpretable, ensuring trust and accountability in high-stakes environments.
The advantages of explainability and interpretability in AI are multifaceted: They provide deeper insights into the decision-making processes of AI models, enabling stakeholders to understand the rationale behind the outcomes. Transparent models enhance accountability by making AI systems interpretable and explainable to human users. This builds confidence in AI systems, fostering fairness, trust and user acceptance.
However, the complexity and opacity of many AI models—particularly those leveraging deep learning models—present significant challenges. These “black box” systems often operate with little or no transparency, making it difficult to apprehend how and why specific decisions are made. This lack of transparency can lead to biased outcomes, raise ethical concerns, and ultimately erode trust. Addressing these issues requires rigorous approaches that make AI systems explainable and interpretable.
This workshop on eXplainable AI (XAI) aims to explore cutting-edge methods, tackle existing challenges and risks, and discuss practical applications of XAI to address the pressing need for transparency and trust in AI systems
By bringing together researchers and practitioners, this workshop aspires to provide an interdisciplinary forum for sharing recent research advancements in XAI approaches, best practices, design principles, open challenges, inherent
risks, and practical applications. The program will feature keynote presentations, open discussions, idea exchanges, and practical, instructor-led hands-on training sessions.
Time | Plenary Sessions |
---|---|
9:00 – 9:15 | Welcome and opening |
9:15 – 9:55 | Prof. Dr. Samek Wojciech, Technical University of Berlin & Fraunhofer Heinrich Hertz Institute (HHI), Berlin, Germany. Component-level Explanation and Validation of AI Models. ABSTRACT |
9:55 – 10:35 | Prof. Wassila Ouerdane, CentraleSupélec, Paris-Saclay University, France. Interpretable Image Classification Through an Argumentative Dialog Between Encoders. ABSTRACT |
10:35 – 10:50 | Break |
10:50 – 11:30 | Dr. Celia Cintas, IBM Research Africa, Nairobi. A Tale of Adversarial Attacks & Out-of-distribution Detection Stories in the Activation Space. ABSTRACT |
11:30 – 12:15 | Dr. Mourad Zerai, ESPRIT School of Engineering, Tunisia. Why Explainability Matters. ABSTRACT |
12:15 – 13:00 | Break |
13:00 – 13:40 | Prof. Alberto Bosio, University of Lyon – Lyon Institute of Nanotechnology. Reliable and Efficient Hardware for Trustworthy Deep Neural Networks. ABSTRACT |
13:40 – 14:20 | Prof. Giuseppe Primiero, University of Milan, Italy. BRIO: A Bias and Risk Assessment Formal Methodology and Tool. ABSTRACT |
14:20 – 15:00 | Dr. Yazan Mualla, University of Technology of Belfort-Montbéliard (UTBM), France. Human-Agent Explainability Architecture: Application of Remote Robots. ABSTRACT |
15:00 – 15:15 | Break |
15:15 – 15:55 | Pr. Farkhund Iqbal, College of Technological Innovation, Zayed University, UAE. The Impact of Explainable AI Models in Enhancing Interpretability and Transparency for Cybersecurity and Digital Forensics. ABSTRACT |
15:55 – 16:35 | Dr. Amit Dhurandhar, IBM T.J. Watson, Yorktown Heights, NY, USA. Explainable AI from Classification to Generation. ABSTRACT |
16:35 – 17:15 | Michael Boone, NVIDIA, USA. Trustworthy AI at NVIDIA. ABSTRACT |
17:15 – 17:25 | Closing remarks |
Workshop Planning*
Morning Session | Afternoon Session | ||
---|---|---|---|
8:30 – 9:00 | Registration & Setup | 13:00–16:00 | Model-specific explainability approaches |
9:00 – 12:00 | Model-agnostic explainability approaches | 16:00–17:00 | LLM Interpretability |
12:00 – 13:00 | 1 hour Break | 17:00–17:30 | Wrap-up and Closing remarks |
Artificial Intelligence (AI) systems have become an integral part of modern society, with their deployment spanning numerous critical applications such as healthcare, finance, energy, transportation, cybersecurity, justice systems, and manufacturing. Many of these AI-driven systems are designed to assist in or directly make decisions that can have far-reaching implications. Hence the need for AI models that are not only accurate but also explainable and interpretable.
The advantages of explainability and interpretability in AI are multifaceted: They provide deeper insights into the decision-making processes of AI models, enabling stakeholders to understand the rationale behind the outcomes. Transparent models enhance accountability by making AI systems interpretable and explainable to human users. This builds confidence in AI systems, fostering fairness, trust and user acceptance.
However, the complexity and opacity of many AI models—particularly those leveraging deep learning models—present significant challenges. These “black box” systems often operate with little or no transparency, making it difficult to apprehend how and why specific decisions are made. This lack of transparency can lead to biased outcomes, raise ethical concerns, and ultimately erode trust. Addressing these issues requires rigorous approaches that make AI systems explainable and interpretable.
This workshop on eXplainable AI (XAI) aims to explore cutting-edge methods, tackle existing challenges and risks, and discuss practical applications of XAI to address the pressing need for transparency and trust in AI systems.
By bringing together researchers and practitioners, this workshop aspires to provide an interdisciplinary forum for sharing recent research advancements in XAI approaches, best practices, design principles, open challenges, inherent risks, and practical applications. The program will feature keynote presentations, open discussions, idea exchanges, and practical, instructor-led hands-on training sessions.
The primary objectives of this workshop are as follows:
Note: Attendance on the first day, whether onsite or online, is free of charge. However, participation in the second day’s practical XAI training requires a registration fee and is conditional upon attendance on the first day.
Email : contact.esb@esprit.tn Tél : +(216) 70 168 700
Powered by Same Team
This presentation discusses what Trustworthy AI (TAI) means, what we are doing, and how we are deploying trustworthiness as an embedded property of technology for us, our customers and partners, and the greater ecosystem.